Season 19 of Grey’s Anatomy premieres later this week, and I am one of the many dedicated fans who has been with the show since Day One. I remember in college, in the pre-streaming era, I bought a cheap VCR for the sole reason of recording Grey’s episodes during my evening chem lab. I can tell you exactly where I was when I watched the bazooka bomb episode guest starring Christina Ricci and Kyle Chandler. This show has been a constant for me over the past 18 seasons (wow!) spanning 17 years (WOW!).
And in these glorious 18 seasons, the Grey’s universe has accumulated an enormous amount of mess among its characters. There are love stories, heartbreaks, one night stands, family drama, spinoff shows, workplace feuds, physical fights, natural disasters, tragedies – I could (and might) go on.
Earlier this summer, I was brainstorming infographic ideas for this blog and was drawing inspiration from the abundance of data out there about The Bachelor franchise. My most popular blog post is a Bachelor data interactive; the Instagram account @BachelorData has a huge following; there is even an entire book about Bachelor game statistics called How to Win The Bachelor. It occurred to me that, with 18 seasons of Grey’s under our belts, there is so much data to extract about Grey’s storylines – plus a huge fan base out there who might find it interesting to nerd out on. Just like there is this huge community in Bachelor Nation, is there perhaps an underserved market of Grey’s dataheads?
So now, three months after I began this research project, and with only a few days left before more romantic entanglements ensue in the S19 premiere, I am extremely proud to present my very first Grey’s data infographic. This is essentially a network diagram which visualizes the various romantic connections (“links”) among the characters (“nodes”). Note that the Grey’s universe is huge, and as such this graphic is best seen in full-screen, zoom-in, high-res mode here.

There are so many different flavors of romantic connections I considered – past vs present, unrequited vs consummated, on-screen vs off-screen. I developed these rules below to determine which relationships were important enough to qualify for this infographic:
What kinds of “nodes” and “links” are eligible to appear here?
- Characters who are eligible to appear as “nodes” must be at least one of the following:
- (a) series regulars with at least 1 romantic link
- (b) 1st degree romantic links to series regulars
- or (c) 2nd degree links to a series regular as long as this person’s 1st degree link is with any Grey-Sloan Memorial (GSM) doctor.
- Characters and any of their eligible connections also must also meet all of the following criteria:
- (a) credited in at least 1 Grey’s Anatomy main show episode
- (b) physically appears in in a “present day” storyline
- and (c) has at least a first name.
- Characters are given a romantic connection if they, at minimum, have either had sex or have a dating history that goes beyond a one-time date.
- The occurrence of two characters’ romantic connection can happen at any point (on-screen or off-screen, historical or future to their appearance, or even in separate spinoff show episodes) — as long as each character at one point appears in a “present day” Grey’s Anatomy storyline.
- Familial links (parent/child or siblings) are shown between characters who have other romantic entanglements, to visualize the family drama also afoot.
What kinds of “nodes” and “links” were excluded?
- Two characters are not given a romantic connection in these scenarios:
- (a) they have only been on one sex-less date (e.g., Derek Shepherd’s awkward date with Sydney Heron)
- (b) they only kiss briefly then one or both parties puts a stop to it (e.g., Jordan Wright kisses Miranda Bailey but she’s having none of it; Alex Karev and April Kepner kiss once, but when he tries to go further she stops him and he storms off)
- (c) one or both characters have romantic feelings but never act on it (e.g., Lexie Grey’s crush on George O’Malley, Taryn Helms’s crush on Meredith Grey)
- (d) one character never appears on a present day Grey’s Anatomy storyline (e.g., Teddy Altman’s late ex-girlfriend Allison who dies in a 9/11 flashback)
- or (e) they are only romantically connected to someone who is not a Grey-Sloan doctor (e.g., Thatcher Grey’s second and third wives).
- If a romantic partner is only discussed but the character never appears present day, this person/link is not shown.
- This “disqualifies” exes who never appear or only appear as flashbacks (e.g., due to estrangement or death) — which unfortunately disqualifies important backstories, like Tom Koracick’s late wife Dana.
- This was a tough decision, but I had to draw the line somewhere. This prevents the chart from getting clogged up any time a character offhandedly mentions an off-screen/historical ex-lover.
- While familial links are shown where they exist, family members are not listed here unless they have a primary or secondary romantic connection to a series regular themselves
And of course I did more than just draw out the connections; I crunched those numbers, too. Here you can see, of the 21 “main characters,” who has the most 1st degree romantic partners (i.e., direct connections) as well as who have the most 1st degree + 2nd degree partners (i.e., your partners plus your partners’ partners).
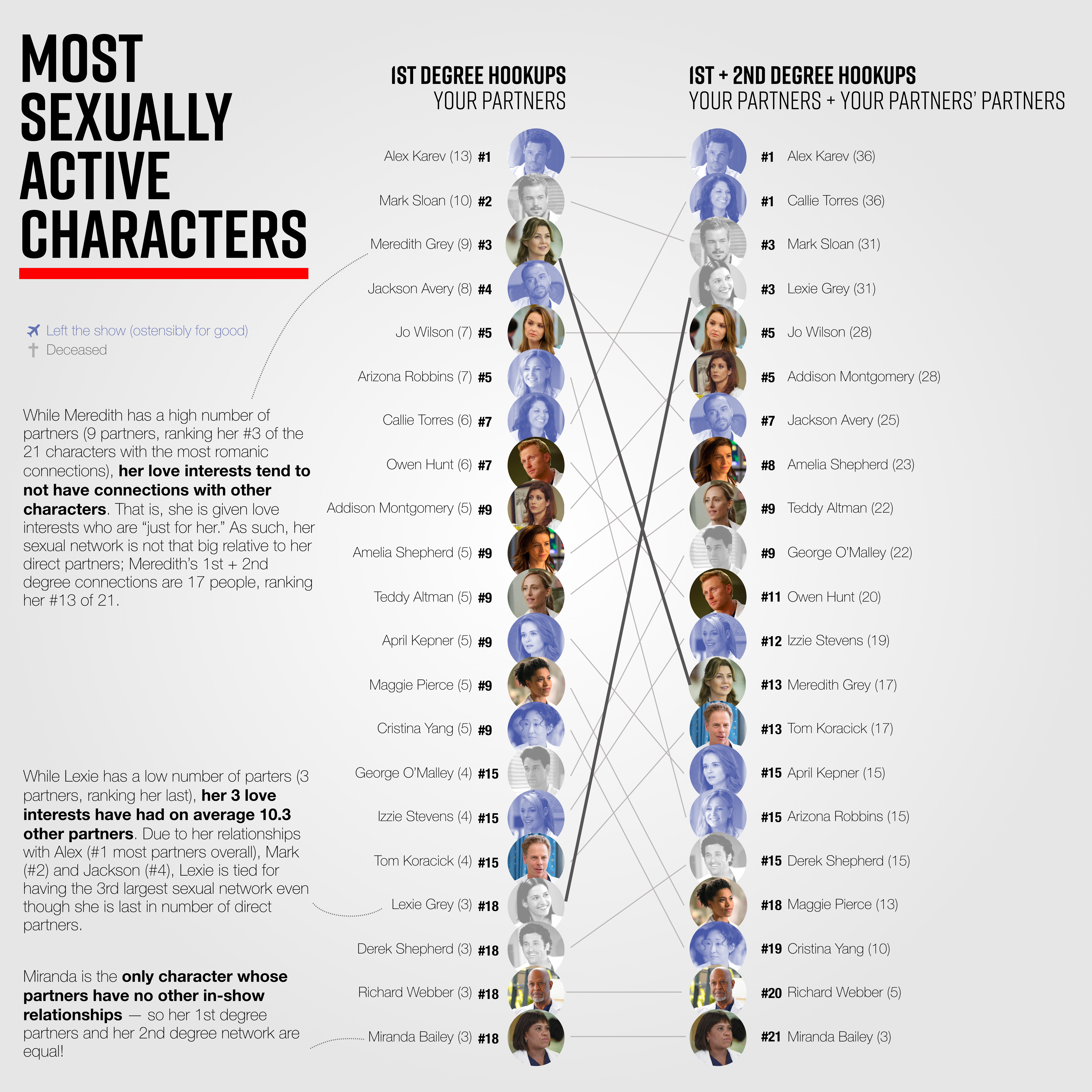
For Grey’s fans, it’s not surprising to see Alex Karev and Mark Sloan at the top of the “most number of partners” ranking. They both were known for being promiscuous around the hospital, and they both were given longer-term romantic storylines. I was pleasantly surprised to see Jo Wilson so high – this means the writers are giving her a complicated personal life (great for her screen time!) and as one of the only main characters who is presently single, I could see her increasing her partner count in coming episodes. Her romantic connectedness also means that anyone who newly becomes linked to Jo will immediately have high drama potential.
One of the most interesting parts of this analysis for me was the “1st + 2nd degree hookups.” This tells us not just how many partners the character had, but how entangled their chosen partners are with other characters on the show. For example: While Meredith is #3 in direct partners, her partners aren’t likely to have relationships with others on the show; as Meredith is the lead, I think this protects her from getting into petty interpersonal drama.
Speaking of protection, Miranda Bailey is completely isolated from the drama. While she is given 3 romantic partners over the past 18 seasons, none of these 3 partners ever have an on-screen romance with anyone else. Bailey is often the moral compass and the authority figure; again, this feels like a choice to protect her storylines and her reputation.
One last thing I made for this analysis: If you follow me (@huskerdana on Instagram), you’ll see I posted a main grid carousel for the first time with an “explainer” of this infographic! Here is the slide show I’m putting on social:
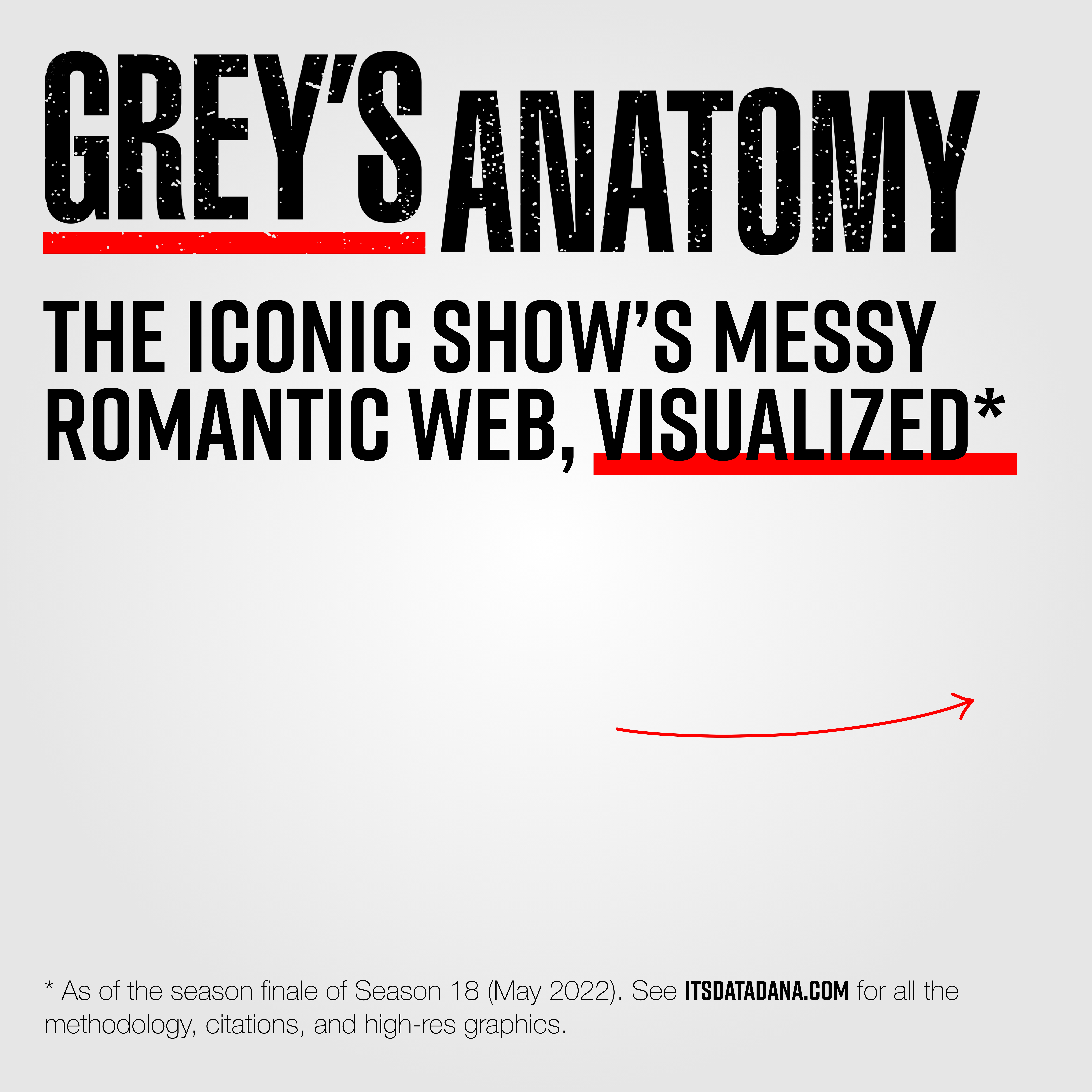
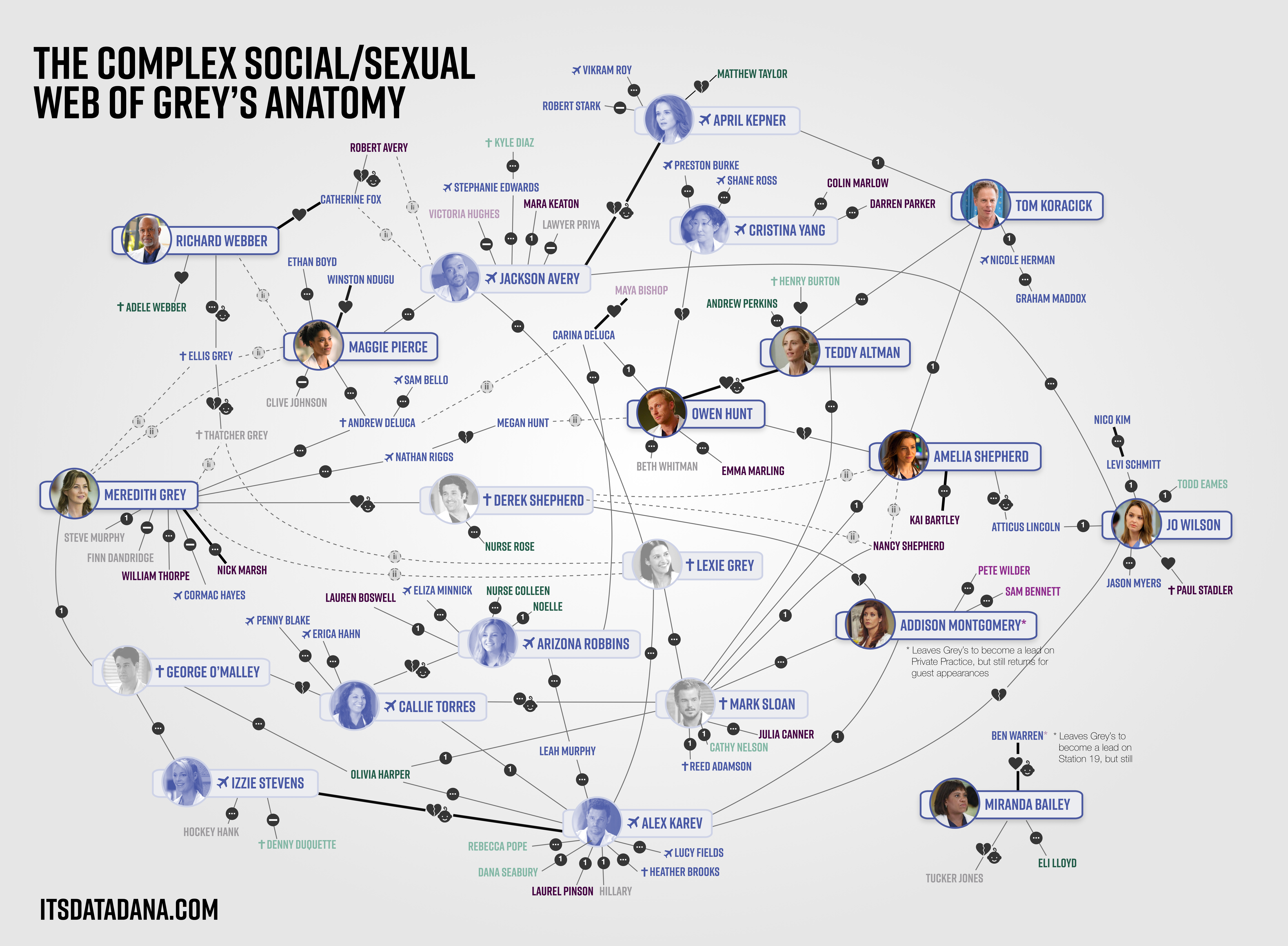
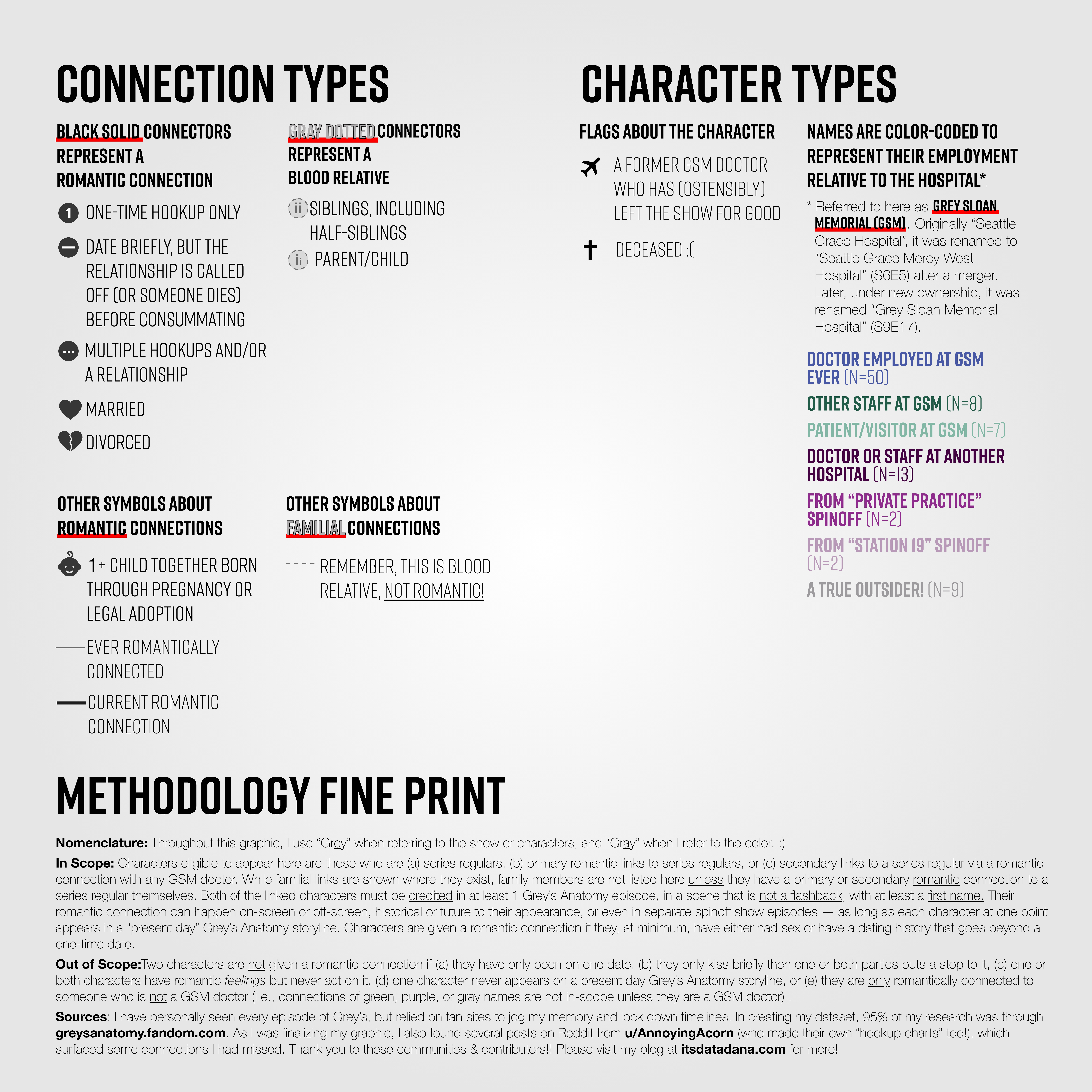
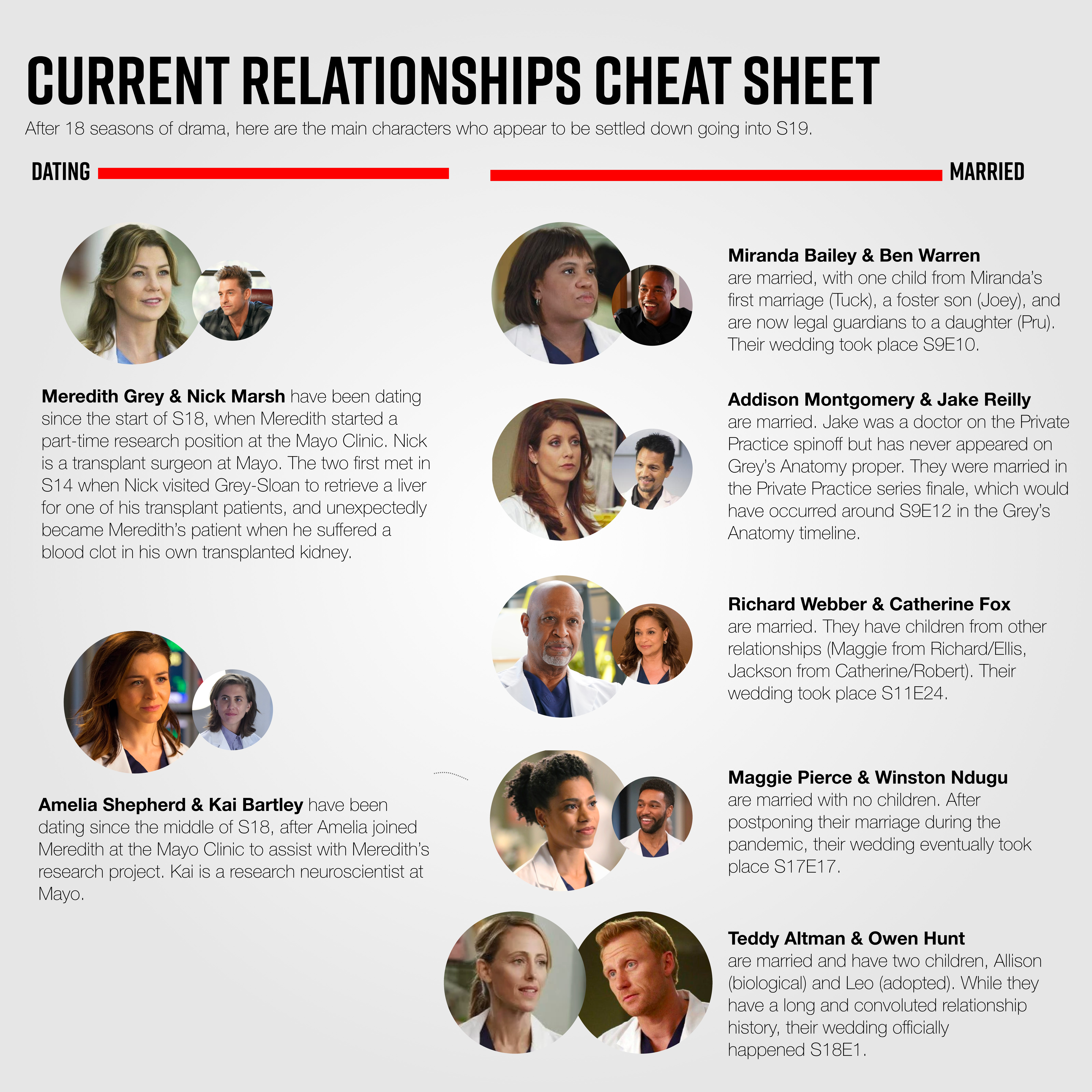
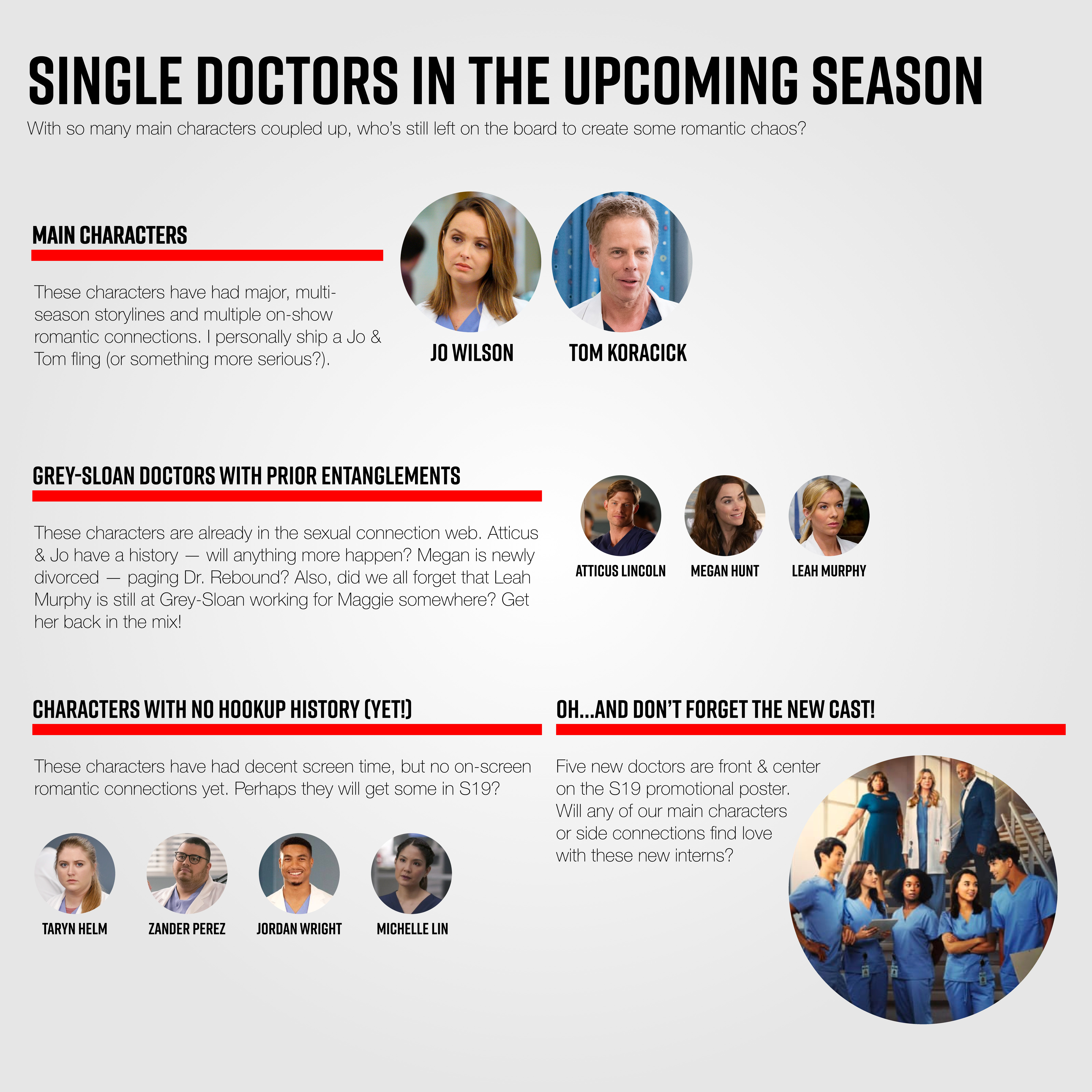
Why have I never broken up my analyses into a carousel like this?! It’s so cute! I’ve been honestly inspired by @BachelorData, who creates fun, engaging, easy-to-share videos and multi-slide posts to really make sure the world sees all the hard work she does to capture data about The Bachelor. Plus I’ve been learning Adobe Illustrator and Adobe XD, so I feel like my toolkit is growing in a really creative way. This entire effort took me several months, and I’m honestly so proud of it. I hope dipping my toes into some new design software & new social media techniques will help this infographic reach as many of my fellow Grey’s enthusiasts as possible.
There is so much untapped potential in this Grey’s Anatomy data, and so much to unpack with my fellow Grey’s dataheads. Should I dive deeper into the romantic connection data? Collect new storyline data points? Quantify the ways in which the show has evolved over time? Comment below!
-Dd